Understanding the Downsides of AI: A Critical Examination
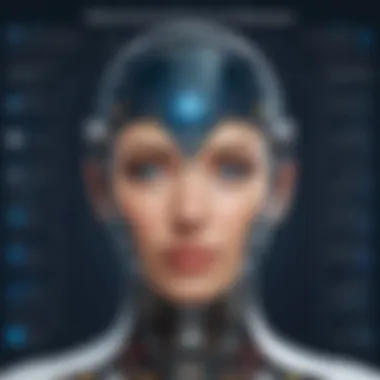
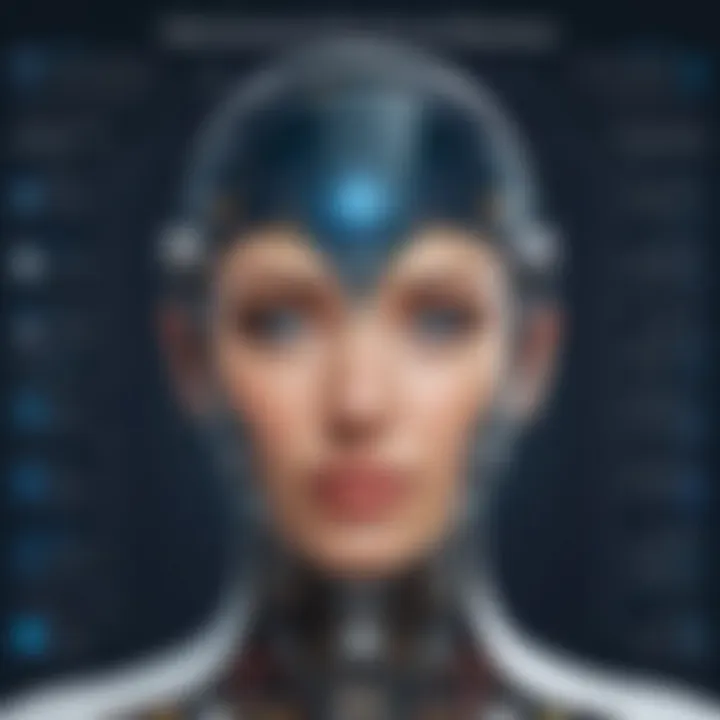
Intro
Artificial Intelligence (AI) is reshaping our world in many ways. It brings forth various benefits but also reveals notable downsides that we must confront. This article unfolds the critical examination into these challenges, offering clarity on issues pertaining to ethics, employment displacement, bias, and security. A deep dive into these topics helps us read between the lines of technology's promise and peril.
Ethical Concerns
AI raises significant ethical dilemmas. One key question revolves around the use of AI in surveillance and data collection. Governments and corporations can harness AI to monitor individuals, which leads to privacy infringements. Furthermore, an ethical discussion is necessary about how AI can impact marginalized communities. Algorithms can perpetuate discrimination when trained on biased data, leading to unfair outcomes in hiring or law enforcement.
Job Displacement
We cannot ignore job displacement, which remains a dominant concern across areas like manufacturing and services. AI technologies can automate tasks previously done by humans, leading to workforce reductions. While AI creates new job types, there still exists a gap as helpless workers may lack skills for these roles. A reassessment of education and skill-building tactics will be essential to manage this transition effectively.
Bias in Data Sets
The bias embedded within AI algorithms stems from historical context. Algorithms learn from existing data, making it crucial to scrutinize the information fed into these systems. Inappropriate training datasets can result in flawed decision-making. For instance, facial recognition technologies often perform unequally across different demographics. Ensuring diverse data incorporation is imperative for the fairness and accuracy of AI outputs.
Security Vulnerabilities
As AI continues to advance, security vulnerabilities pose a significant risk. Cyber criminals can exploit weaknesses in AI systems, leading to potential breaches in personal data or infrastructure. Moreover, AI-powered tools can automate attacks, making threats harder to identify. Institutions must implement robust security measures and remain agile in an ever-evolving cyber landscape.
Autonomous Decision-Making
Finally, the sensitivity surrounding autonomous decision-making brings numerous challenges. Self-driving vehicles and automated financial trading algorithms demonstrate the capacity for making choices without human intervention. However, this autonomy raises pressing questions about accountability. When mistakes occur, identifying layers of responsibility becomes increasingly complex.
To encourage informed discussions, the complex relationship between AI and society must be understood.
In sum, understanding the nuances of AI necessitates thoughtful engagement with its downsides that include ethical, social, and economic implications. As technology advances, maintaining scrutiny over these challenges can help shape a balanced approach toward future developments.
Foreword to AI and Its Pervasiveness
Artificial Intelligence (AI) is intruding in much aspects of human life and infrastructure. From recommending products to automating processes, AI's influence touches various industries. Its capability to process large data sets quickly and effectively provides efficiency and enhanced productivity. Furthermore, AI algorithms continuously learn from their environments, leading to more tailored experiences for users.
However, while exploring its pervasive nature, one must also consider various implications it carries. AI can optimize systems, create efficiencies, and offer powerful predictive insights. The benefits can elevate businesses and improve consumer experiences. Yet, the reliance on these technologies raises questions. How do they affect employment? Privacy? Ethical standards?
The significance of AI knows many dimensions. Its ability to reshape the job market through automation is noticeable already, pushing for changes in workforce dynamics. Education and reskilling become crucial responses to these transformations.
Moreover, the ethical considerations associated with AI cannot be overlooked. As machine learning systems learn from biased data, issues of fairness arise. They can impact society in profound ways—determining probabilities for loan approvals, even influencing judicial outcomes.
It is critical that understanding AI’s advance involves not only recognizing its capabilities but also acknowledging its potential downsides. Each example of its use—be it in healthcare, finance, or social media—carries its questions on accountability, bias, and security.
Understanding AI Technology
Understanding AI Technology
The exploration of AI technology is critical in comprehending the wider implications of artificial intelligence. By understanding the technological foundations, readers can analyze its potential, benefits, and associated risks with greater clarity. The role of AI spans many sectors such as healthcare, finance, and education. Each sector uses AI differently, including applications in diagnostics, fraud detection, and personalized learning systems. Increased awareness of how AI functions serves not just technological minds but also those making policy decisions regarding its deployment.
This section will address how AI evolved and its current landscape, emphasizing key facets of its application and impact. An in-depth perspective on understanding AI technology allows for recognizing risks, especially regarding ethics and bias,: guiding the discourse toward responsible utilization.
Historical Context of AI Development
AI's story is a narrative that spans decades. It began in earnest during the mid-20th century. Early pioneers like Alan Turing and John McCarthy conceptualized machines capable of simulating human intelligence. Initially, the focus was on symbol manipulation and problem-solving.
Through in-depth research and experimentation, AI has undergone various phases:
- The 1970s wave of optimism leading to significant government funding faltered in 1980s over expectations that were not met.
- Innovations in computing power and data availability re-ignited interest in the late 1990s known as the AI Spring.
Modern AI technology relies heavily on machine learning, enabling computers to learn patterns from vast datasets. This iterative learning contrasts sharply from early AI's rule-based systems. Today’s systems, particularly profoundly neural networks, can perform tasks that once seemed exclusive to human capability. The context expands upon our understanding significantly, setting a suitable stage for evaluating its current influence.
Current Applications of AI
AI applications today are numerous, impacting everyday life subtly yet profoundly. It is crucial to identify common use cases that showcase AI's pervasive influence. Some include:
- Healthcare: AI systems are refining diagnostic processes, predicting patient outcomes, and personalizing treatment plans by analyzing extensive clinical data.
- Finance: In fraud detection, algorithms sift through transaction patterns to identify anomalies faster than a human could; in investment analysis, AI predicts market trends.
- Education: Intelligent tutoring systems adapt to students’ learning styles, offering personalized resources and feedback to enhance the learning experience.
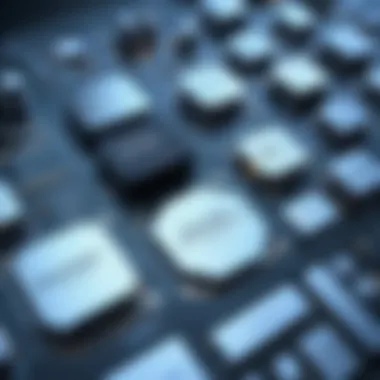
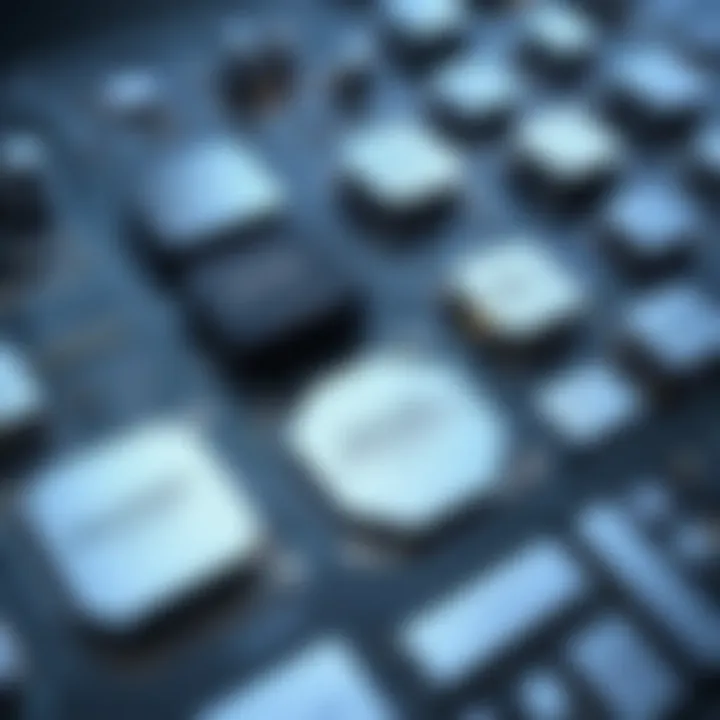
These deployments reveal a march toward sophistication in technology. They have sparked excitement about the promises AI holds in revolutionizing mundane or complex tasks. However, with these advantages come new risks—specifically ethical concerns related to privacy, accountability, and fairness. As this continent advances, understanding both applications and implications stays paramount in promoting a balanced dialogue around AI evolution.
Ethical Considerations
The discussion around Artificial Intelligence is incomplete without addressing ethical considerations. As AI technologies become intricately woven into the fabric of society, understanding these ethical implications is crucial. The systems shaped by AI are not only programmed to execute tasks but also shape decisions and influence biases within institutions. Thus, newly arising ethical challenges require critical evaluation and careful governance.
Privacy Issues in AI Systems
Privacy is a paramount concern in the realm of AI. In a world where personal data is the currency of digital interaction, the role AI plays in managing sensitive information raises significant questions. Various industries employing AI rely heavily on data collection. For its capabilities, a vast amount of individual data is often harvested. Examples include personalized services in social media platforms like Facebook, e-commerce transactions, and health data analysis. A report by en.wikipedia.org highlights that algorithms like those used in consumer behavior prediction must be scrutinized since they can potentially infringe on individual privacy.
With such systems in place, maintaining user confidentiality is a persistent challenge. It is challenging to ensure appropriate data protection measures, as end-users often lack visibility on how their data is collected and utilized. This invisibility can lead to cases of misuse or data leaks, potentially causing severe reputational damage and violation of trust. The implications of data mishandling extend beyond individual breaches; they can, indeed, compromise the ethical integrity of organizations and AI developers alike.
The Problem of Accountability
As AI continues to evolve, the issue of accountability grows increasingly complex. Who is responsible when an AI system makes an incorrect decision or generates harmful outcomes? This question vexes researchers and policy makers alike. Traditional frameworks of accountability, usually anchored in human actions, encounter understability complications in the mechanical decisioning by AI systems.
An alarming instance is the application of complex algorithms in law enforcement, often scrutinizing citizens based on predictive policing methods. This leads to uncomfortable legal and societal questions about accountability. Conculding who bears the consequences of AI-driven errors—for instance, wrongful arrests or misleading charges—poses challenges for ethical policy terrains. An emerging thought in governance circles is that clear regulatory frameworks must be developed to ensure not only transparency but also accountability standards in AI operation.
Clarifying responsibility involves not only delineating roles within organizations but also necessitates interdisciplinary collaboration across legal, technological, and ethical fields. This is not an easy task, yet it must be recognized as essential as AI technologies become more prevalent.
Accountability in AI is a core issue that needs consistent re-evaluation to adapt to the shifts in both technology and societal expectations.
Job Displacement and Economic Impact
The discourse surrounding AI cannot ignore the impending consequences instigated by automation on the workforce. Understanding job displacement is vital in this conversation. This is not merely about the loss of specific jobs but rather the enduring shift in the economic landscape caused by AI adoption. Many experts agree that low-skill jobs face higher risks of automation, while positions requiring advanced cognitive skills might transform rather than disappear.
What is noteworthy is that AI's capacity to streamline operations often collapses traditional concepts of labor. Staff that once managed straightforward tasks can find themselves obsolete, leading to profound socio-economic consequences. The economic landscape may shift as job sectors adjust to these continual advancements. This dynamic evolution can lead to economic discrepancy, such as income inequality, creating a paradox where technologies designed to liberate individuals might actually subjugate them financially.
The Future of Work in an AI-Driven World
Future scenarios in an AI-centered workplace are rife with uncertainty yet they provide a fascinating case for study. While enhancement in productivity is a predictable outcome, perceiving the labor landscape's complete alteration is crucial. Many predict swelling growth in sectors like technology and healthcare as AI-integrated workflows thrive.
Moreover, the gig economy might evolve to encompass more atypical roles or create novel positions altogether, requiring human creativity alongside machine efficiency. It is essential to chart an understanding of how job functions may pivot from direct ‘doing’ to higher-order functions like managing and interpreting results driven by AI tools. This phenomenon heralds potential benefits, such strengthening lifestyle flexibility and offering varied professional development opportunities.
Reskilling and Upskilling the Workforce
Transforming with technology demands understanding the recourse necessary to navigate these changes. Reskilling and upskilling become pressing measures to ensure individuals remain relevant in an economic landscape significantly altered by AI. While opinions about efficient reskilling methods vary, one consensus lays in promoting adaptability. Prerequisite skills needing emphasis include digital literacy, critical thinking, and emotional intelligence.
Organizations face stiff challenges providing retraining programs. Viable strategies may include:
- Collaborating with educational institutions for holistic training programs.
- Creating hybrid training frameworks combining theoretical knowledge with practical assessments.
- Implementing mentorship schemes matching experienced workers with less experienced ones.
A collective societal effort is essential in upheaving existing educational paradigms emphasizing technical knowledge. Emphasizing interdisciplinary training can foster a society equipped to embrace the demands of an AI-enriched environment, ultimately positioning workers toward sustainable career paths.
Bias and Fairness in AI
The significance of Bias and Fairness in AI warrants careful consideration in light of AI’s wide use. These issues serve as critical indicators of whether AI technologies can be deemed effective and trustworthy. When AI systems include bias, not only does it influence their decision-making processes, but it can also lead to harmful outcomes for individuals and communities. Understanding the dynamics of bias, essential to recognizing its root causes, can facilitate more equitable AI designs.
Origin of Bias in AI Datasets
Bias usually begins in the data utilized to train AI systems. If these datasets do not adequately recognize diversity or represent different demographics, the algorithms reflect these inaccuracies. They can blend false perceptions about certain groups into social norms. Numerous factors contribute to bias in data origins:
- Historical Prejudice: Past discrimination may find its way into datasets. For example, employing historical political data can perpetuate biases rooted in previous injustices.
- Data Selection: The manner in which data is collected often skews its fairness. If a dataset includes data predominantly from certain geographic areas or socio-economic backgrounds, the model may not perform effectively for individuals outside those groups.
- Sampling Processes: Ways of gathering samples can also contribute to bias. Surveys that access only internet users may exclude people with limited access to technology, rendering the dataset insufficiently representative.
In these scenarios, AI can act as a mirror, reflecting existing societal biases rather than helping to rectify them. As such, the need for diverse AI training datasets becomes clear.
Consequences of AI Bias
The repercussions of biased AI systems can be far-reaching, generating negative impacts on various facets of society. Here are two key consequences worth noting:
- Reinforcement of Inequalities: AI can perpetuate social inequalities present in historical data. For instance, biased hiring algorithms imitating existing workplaces may prefer candidates belonging to specific demographics over others, thereby marginalizing deserving candidates.
- Legal and Reputational Risks: Organizations utilizing skewed AI systems confront not only ethical dilemmas but also legal consequences. A biased facial recognition system that inaccurately identifies individuals based on incorrect racial representations raises scrutiny, potentially leading to lawsuits and reputational damage.
In this overcrowded landscape of technology, fairness should not merely be an afterthought but a design principle, inherent to AI development processes.
Security Vulnerabilities of AI Systems
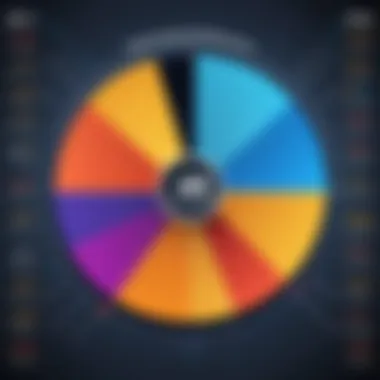
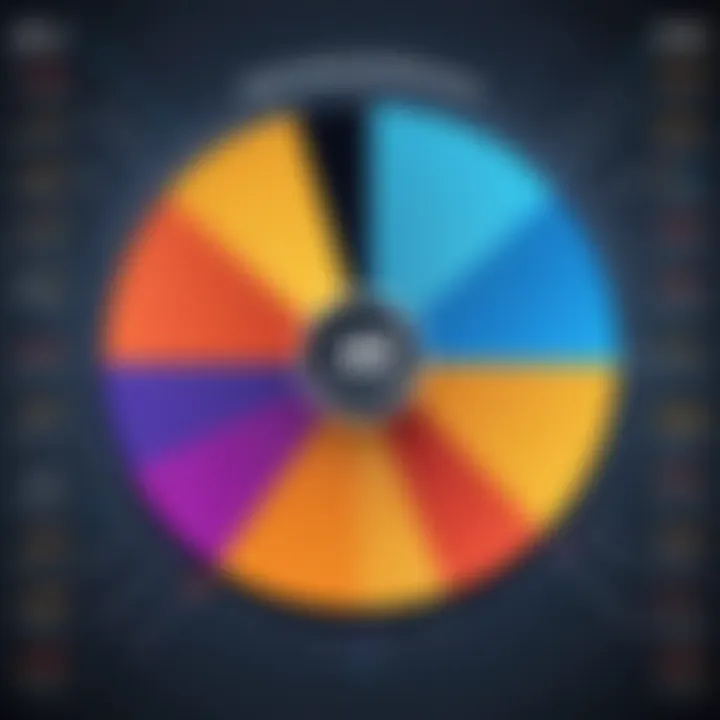
Security vulnerabilities in AI systems are increasingly important as artificial intelligence becomes more prevalent in various sectors. As AI technology evolves, so do the risks associated with its deployment. This article will explore not only how AI tools can enhance security but also their inherent weaknesses, which may be exploited by malicious actors. Understanding these vulnerabilities is essential in creating robust AI systems that can safeguard our society.
AI in Cybersecurity
AI's role in cybersecurity is complex. On one hand, AI systems analyze vast data sets. They detect and respond to threats in real-time, which significantly improves the speed and efficiency of cybersecurity measures. AI can identify patterns and anomalies faster than human analysts, enabling organizations to defend against attacks before damage occurs.
However, the use of AI in cybersecurity also comes with specific risks. Cybercriminals are leveraging AI to make their attacks clandestine and more effective. Integrating AI into cybersecurity does not eliminate vulnerabilities; instead, it transforms them. There are AI models themselves that can be manipulated to evaluate fake signals as genuine, leading to improper responses from the systems.
In addition, many algorithms learn from unfiltered data that could contain biased or irrelevant information. This learning process might generate weak points bottle-necked in machine vulnerabilities like, Spoofing or Phishing campaigns, manipulating AI to access sensitive information.
Threats Posed by Malicious AI
The deployment of malicious AI has developed into a legitimate concern. Unlike human attackers, intelligent algorithms can operate continuously without fatigue, capitalizing on learning capabilities to evolve strategies for sophisticated attacks. Here are some potential threats posed by malicious AI:
- Automated Attack Tools: With the assistance of AI, attackers can automate their offensive tactics, conducting a vast number of actions simultaneously which exceeds any human capacity.
- Data Poisoning: Attackers may inject conflicted information into training sets for AI models. If successful, the integrity of the resulting AI systems can be compromised, enabling false conclusions.
- Deepfake Technology: AI can produce hyper-realistic fake videos or audio, which could mislead viewers regarding real events or individuals. This could influence trust in media and organizations.
Adopting stronger safeguards and infrastructure adaptation strategies can contribute to reducing these vulnerabilities. Improved internal safeguards must go hand in hand with implementing more rigorous testing protocols. All precautionary steps enhance our defenses in this ongoing daily battle between security challengers and AI technologies.
Simply put, while AI adds robust capabilities to security measures, it also brings complexities that necessitate dedicated protections to counteract malicious utilization. Finding equilibrium between fortifying security and using cutting-edge AI remains vital.
Autonomous Decision-Making Challenges
Autonomous decision-making systems represent a pivotal advancement in artificial intelligence. With these systems, machines can make choices with little human intervention. They are capable of complex analyses, analyzing large datasets to produce outcomes that humans may overlook. However, this independence raises questions. Specifically, what ethical considerations arise? What oversight must exist to ensure these decisions are appropriate?
Ethical Dilemmas in Autonomous Systems
The rise of autonomous systems brings forth numerous ethical dilemmas. Algorithms often operate on pre-set rules or learn from data sets. But these data sets can contain biases, leading to harmful outcomes. Decisions made in areas like healthcare or criminal justice reflect these dilemmas. For example, an AI based on biased historical data might discriminate against certain demographic groups, leading to unfair legal penalties.
Furthermore, ethical transparency becomes vital. Operators of autonomous systems might not always understand the underlying algorithms. This ambiguity can create situations where accountability is obfuscated. Who is responsible when an autonomous driving system crashes, or an AI diagnostic machine makes an erroneous suggestion? The reliance on these systems without clear accountability plans could generate distrust and resistance from the public. Thus, when establishing autonomous decision-making protocols, companies and developers must prioritize ethical considerations.
Implications for Human Oversight
The integration of autonomous systems into critical areas makes human oversight essential. Automation provides efficiency, meanwhile, it places significant faith in technology. Solely depending on machines can limit necessary scrutiny. Key areas warrant continuous human involvement to preventing cascading failures.
Decision-making without human intervention postpones essential checks and balances. Having informed personnel review and assess decisions can reinforce accountability and ensure system alignment with human values. This monitoring can be both mechanical and procedural—human operators need to bring context into situations involving ethical complexities.
- Implementing a layered oversight structure can help alleviate concerns. Consider:
- Collaboration between AI and human oversight through system checks.
- Engagement within small teams to evaluate AI outputs.
- Regular training for staff in ethics surrounding AI deployment.
Effective human oversight diminishes risks associated with autonomous learning. It leads teams to be proactive, not reactive, thereby creating smoother integrations of AI systems into workflows.
Societal Impacts of AI Deployment
The integration of Artificial Intelligence into society has fundamental implications that transcend mere technical advancements. The ongoing deployment of AI can significantly reshape all areas of everyday life. Most importantly, it influences social structures, erodes traditional roles, alters values, and transforms interpersonal dynamics. Understanding these societal impacts is pivotal, as AI continues to evolve and change rapidly. Thoughtful reflection on how AI interacts with social aspects will more effectively adapt technological initiatives while safeguarding public interests.
AI and Social Inequality
One prevailing concern regarding AI exists in its potential to exacerbate social inequalities. AI systems, if not designed inclusively, can reinforce existing biases and create disparities in access and opportunities. For example, algorithms guiding loan approvals or job recruitment often rely on data that reflects historical inequities. If this data has bias, those biases can become entrenched in the algorithms themselves.
- Employment market: Widespread automation can eliminate lower-skilled jobs, disproportionately affecting underprivileged populations.
This may lead to a widening gap between those with technological proficiency and those without. Addressing this issue may involve policies encouraging transparency in algorithms and strategies to integrate Fairness into AI applications.
“AI can be a force for good or a vehicle of exacerbation, depending on our choices and those who design the systems.”
Furthermore, education and access to technology remain crucial. Ensuring all sectors of society have equal opportunity to learn necessary skills can help mitigate these dynamics.
Impact on Decision-Making Processes
Artificial Intelligence has a profound influence on decision-making across various spheres, including government, business, and daily life. As more decisions become influenced by AI algorithms, the question arises: who is responsible for the outcomes?
- AI in Governance: Trust in smart systems for public administration could escalate above human-driven governance but relying heavily on algorithms raises moral dilemmas. Misestimates from AI models can lead to flawed public policies.
On an individual level, automated decision-making can subtly infiltrate personal choices, from media consumption to partner selection. This might063516313313713 develop strong societal norms influencing these undoubtedly fluid dynamics.
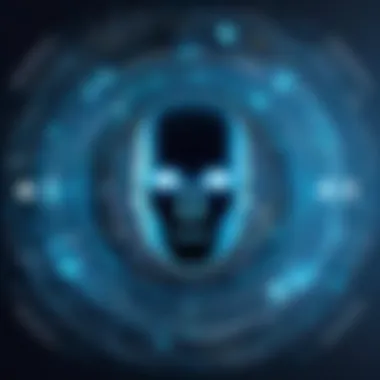
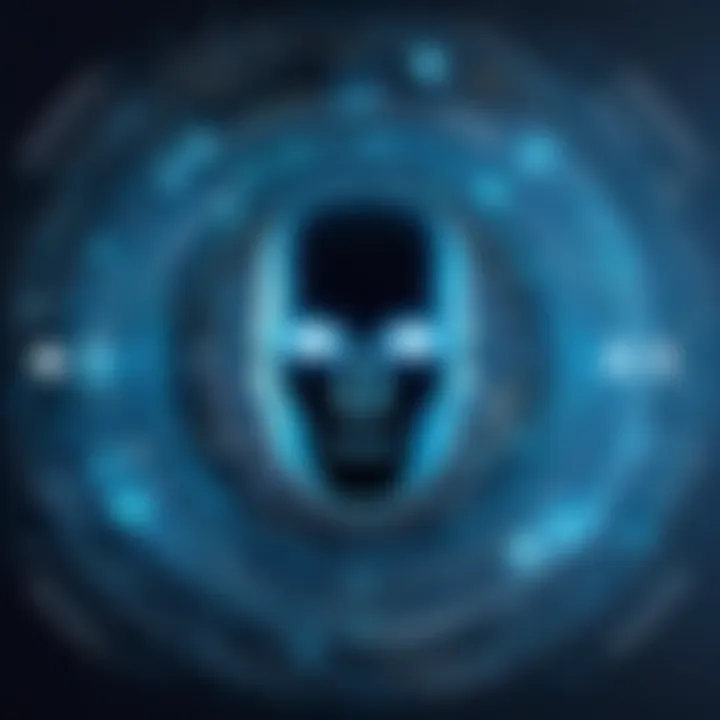
Furthermore, the adoption of AI in data-driven decision-making can diminish the role human judgement holds. While algorithms process information at scale, they lack emotional intelligence, potentially alienating users.
- Ethical framework interstate management: Investing in responsible AI design prompt discussions of ethical considerations in decision-making.
Recognizing predictabilty and balancng competence of machines with human abilities is essential to minimize unintended repercussions.
Regulatory Landscape and AI Governance
The Regulatory Landscape and AI Governance forms a crucial aspect in understanding the overall impact and future trajectory of artificial intelligence. These regulations serve as frameworks to address the significant challenges AI technology brings. They influence not only how AI works but also its societal acceptance and ethical deployment. This subject is not merely academic; it can shape public policy, industrial standards, and user trust in AI systems.
Proper governance in AI ensures that such technologies are developed and deployed responsibly. Insights gained through regulations enable the mitigation of risks associated with automated processes, often fraught with ethical dilemmas. The balancing act for policymakers involves maintaining innovation and protecting fundamental human rights.
Current Regulations Affecting AI
AI is governed by a patchwork of regulations that vary greatly by country and even by industry within nations. Regulations are essential for overseeing data usage, protecting privacy, and ensuring accountability in AI applications. Their role is increasingly significant as businesses integrate more AI tools into operational workflows.
Key Regulations to Consider
- General Data Protection Regulation (GDPR): In Europe, this regulation imposes strict guidelines on how personal data must be handled. Companies utilizing AI have to ensure their processing of personal information fulfills GDPR standards.
- Health Insurance Portability and Accountability Act (HIPAA): In the healthcare sector, AI systems that handle patient information must adhere to HIPAA regulations, which safeguard sensitive health data.
In the absence of universally accepted standards, companies often develop internal mechanisms for compliance. The challenge lies in maintaining a robust yet flexible framework to accommodate rapid advancements in AI. As legal opinions evolve, businesses engaged in AI will need to stay updated to remain compliant.
Future Directions for AI Governance
As the stakes rise, ideas for future governance are being discussed actively among policymakers and practitioners. Creating flexible frameworks that adapt to technological change will be vital. One proposed direction focuses on developing more uniform regulations across jurisdictions, aiming for international standards on AI use.
Potential Areas of Development
- Ethical Guidelines: Performing assessments on emerging technologies will ensure ethical implications are taken seriously. Regulatory bodies can guide organizations to make compliance more than just legal—turning it into an ethical benchmark for operations.
- Accountability Mechanisms: Establishing clear accountability paths for decision-making in AI incorporates safeguards essential for human oversight. This evolution aims to clarify roles in cases where AI systems cause negative outcomes.
In the discourse of AI governance, it's vital that voices from various stakeholders, including experts, companies, and citizens, are considered. Ultimately, Regulation Landscape and AI Governance must pave a responsible technological future, allowing innovation without compromising human rights and ethical standards.
"The dual nature of AI demands thoughtful regulation, steering innovation while mitigating risks."
The Future of AI: Risks and Opportunities
Understanding the future of AI is essential for gauging its potential impact on society, technology, and various industries. As organizations continue to adopt AI solutions, it is crucial to evaluate both the benefits they can offer and the risks they bring along. The balance between these two aspects will determine the trajectory of AI development and its acceptance among different stakeholders.
Balancing Innovation and Ethics
Innovation in AI technology poses both reward and risk. As advanced algorithms and machine learning models emerge, they can bring improvements in efficiency, productivity, and decision-making. However, ethical complexities are always present—notably regarding data privacy, autonomy, and potential bias. Technology must not only progress but also respect core ethical guidelines.
To achieve meaningful growth, organizations must consider incorporating ethical undertaken decisions into the design and implementation of AI systems. Investing in training that educates developers, stakeholders, and users about ethical dilemmas is a vital step in this direction. By fostering discussion around ethical implications from the start, we can better prepare AI strategies that are more transparent and fairer for society.
Key Considerations:
- Integration of ethics into AI design.
- Transparency in decision-making processes.
- Engagement with diverse communities for varied perspectives.
Fostering Responsible AI Development
Responsible AI development emerges as a robust response to the challenges faced from rapid AI advancements. Organizations must adopt policies that promote these unfinished practices, ensuring recognition of ethical dilemmas associated with AI.
Effective frameworks governing AI include guidelines denoting fairness and accountability, regularly reviewing these systems. Moreover, employing interdisciplinary teams for diverse viewpoints can lead to more responsible solutions. Particular attention should focus on adequate risk assessment protocols which highlight safety critiques before implementing AI technologies.
Strategies for Responsible Development:
- Encourage cross-disciplinary collaboration.
- Emphasize robust assessment for fairness and accountability.
- Document and share practices widely to cultivate a knowledge base.
“Technology should complement humanity’s needs, rather than compromise our principles.”
The End: Navigating the Downsides of AI
In the age of advanced technology, the importance of understanding AI's downsides cannot be overstated. As we have seen throughout this article, AI affects many aspects of our lives, from how we work to our ethical frameworks. Highlighting the critical points allows us to ground discussions in sober analysis rather than sensationalism.
Key aspects of navigating these challenges include:
- Awareness of Ethical Concerns: Innovative technologies should come with ethical reasoning. A clear understanding of privacy issues and accountability can navigate proper use.
- Addressing Job Displacement: The conversation must shift to involve reskilling individuals affected by automation. A focus on workforce development ensures future job markets are adaptable.
- Mitigating Bias in Algorithms: Recognizing the sources of bias, such as faulty datasets, is crucial. Developing and implementing corrections can help create fairer AI applications.
- Enhancing Security Measures: As vulnerabilities become more apparent, investing in robust security within AI systems becomes vital.
- Balancing Autonomy with Oversight: The dual need for autonomous systems and human oversight should guide AI design and deployment.
Fostering responsible AI development is essential for ensuring its benefits complement our societal well-being. This sustainable vision requires the stakeholders to actively participate in shaping regulations and industry standards relevant to AI technology.
"A robust framework for AI technology in practice rests on a nuanced understanding of its implications on society, far from a simplistic appreciation of its capabilities."